AI and Fintech: Guide to AI Risk Management for Fintech Leaders
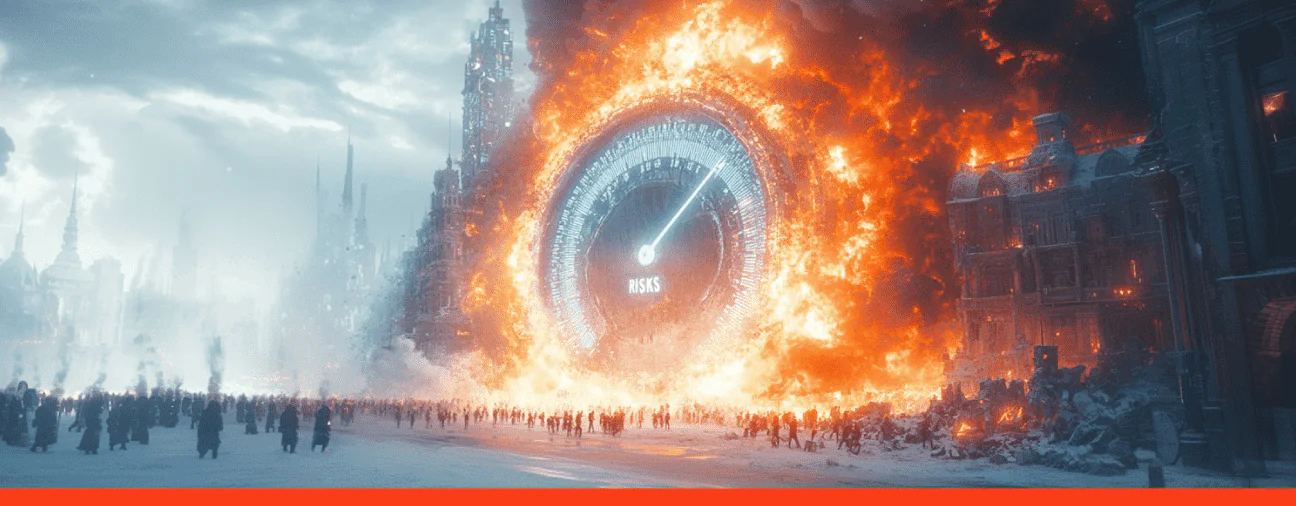
It usually starts with a win: an AI model that cuts fraud losses in half, speeds up credit decisions, or improves onboarding with fewer human touchpoints. Encouraged by early results, teams scale quickly until something quietly goes wrong. A flagged user can’t understand why they were denied access. A regulator asks for an audit trail that doesn’t exist. A drifted model starts approving the wrong kind of risk.
This isn’t a hypothetical scenario. It’s the pattern many fintechs are already facing. The pressure to move fast with AI is real, but so is the cost of treating it as a black box or a side project. In this article, we take a clear-eyed look at the risks that don’t always show up on dashboards, from model degradation and data leakage to strategic over-reliance. As a company that provides fintech AI development services, we’ll help you evaluate your organization’s readiness to manage them and offer practical guidance on how to prevent small oversights from becoming systemic problems.
AI maturity shapes your risk profile — here’s how
Fintech artificial intelligence is rarely deployed all at once. Most organizations move through stages: starting small with automation, scaling gradually, and eventually building AI into core operations and infrastructure. But here’s what many teams overlook: as your AI in financial technology capabilities grow, so do your risks, and they become more complex, less visible, and harder to reverse.
A useful way to frame this evolution comes from Gartner’s Finance AI Maturity Model, which outlines five stages of AI adoption, from minimal experimentation to full strategic transformation. At each phase, new risks emerge, not only technical but operational, regulatory, and ethical.
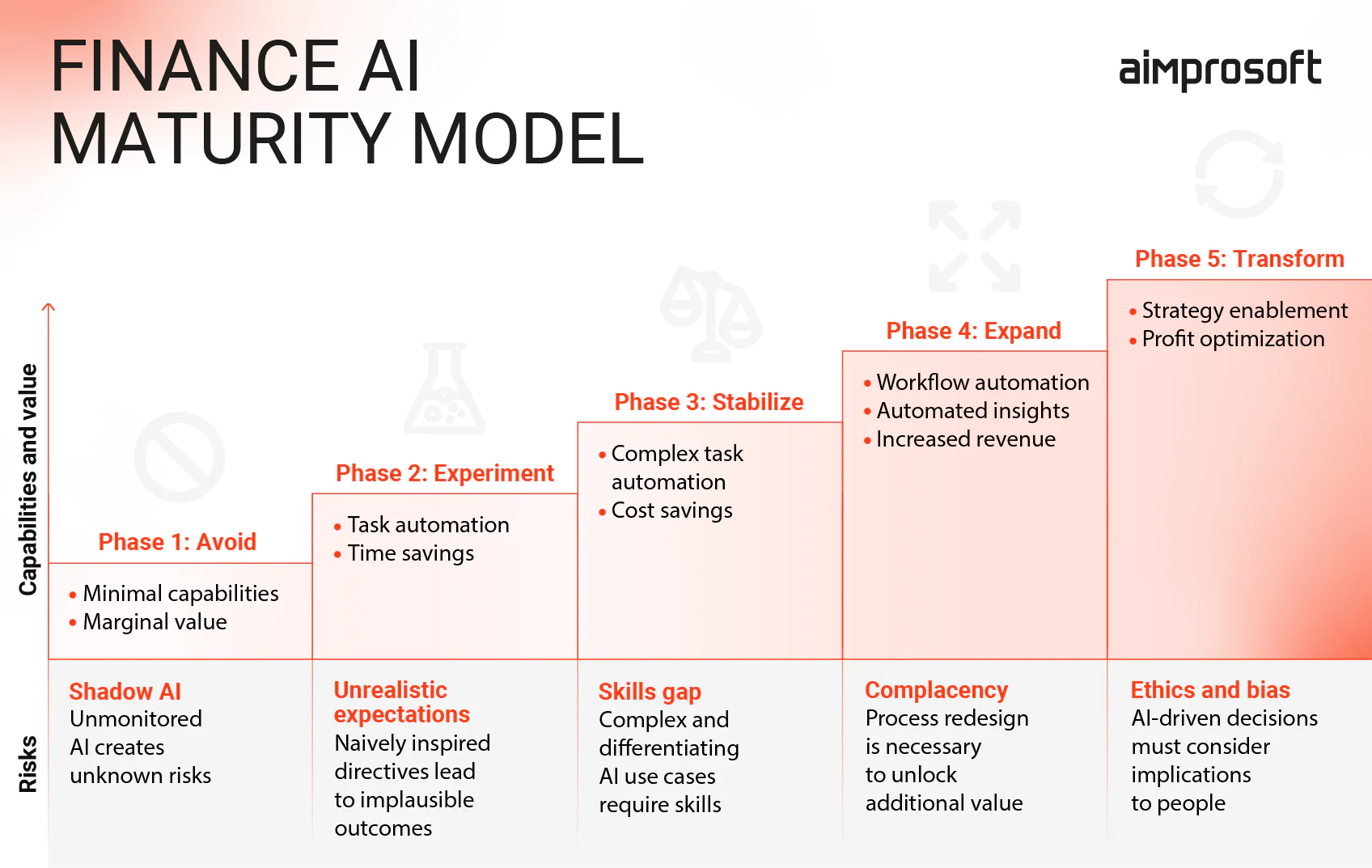
Finance AI maturity model
Let’s look at this model through a fintech lens and explore what you need to watch for at every stage.
Phase 1 – Avoid: The silent threat of Shadow AI
Fintech organizations in this stage haven’t formally merged AI and fintech. However, that doesn’t mean AI isn’t present. Shadow AI emerges when teams experiment with fintech AI tools (like LLMs or decision models) without oversight, leading to unmonitored systems making business-impacting decisions. Even if your official policy says “no AI,” your risk exposure may already exist through marketing tools, plug-ins, or auto-scoring models embedded in vendor platforms.
Phase 2 – Experiment: Risks of overpromising and underbuilding
Early experimentation often leads to unrealistic expectations. You may expect fully automated systems after POC demos, while teams may lack the depth to assess model robustness, data sufficiency, or hidden bias. This disconnect often results in unvalidated models being deployed too soon, public data being used without proper security measures, and critical decisions being made based on outputs that can’t be traced or explained.
Phase 3 – Stabilize: Where complexity outpaces capability
At this point, AI is operational, which may be used for automating underwriting, fraud scoring, or portfolio segmentation. But success breeds risk. As a fintech company, you may encounter a skills gap: the AI is running, but no one on the team knows how to monitor drift, retrain the model, or explain decisions to auditors. This phase introduces technical debt that can’t be easily undone. Without MLOps, robust validation pipelines, and monitoring infrastructure, the AI layer becomes a liability.
Phase 4 – Expand: Complacency is the costliest risk
With multiple models deployed, some fintechs fall into process inertia, assuming systems will self-sustain. But AI for fintech doesn’t age gracefully. Regulations shift, data distributions evolve, and what was accurate last quarter may now be biased or broken. Performance dips go unnoticed, compliance teams aren’t looped in, and retraining schedules become irregular. This is where missed risks get expensive quite fast.
Phase 5 – Transform: Ethical risks and long-tail exposure
At this level, an AI fintech solution is embedded into core decision-making, credit policies, product eligibility, and pricing logic. Now, the risks are deeply strategic: reputational damage from bias, systemic compliance failures, and ethical fallout from opaque decision-making. Boards and regulators will increasingly expect model explainability, audit trails, and governance frameworks, and the lack of these can halt expansion or trigger investigations.
Understanding where you are on the AI maturity curve is crucial, not only for measuring progress but also for anticipating the next risks. In the following sections, we’ll break down the core categories of risks of artificial intelligence in fintech that you should actively prepare for as part of a proactive fintech risk management strategy, and how to mitigate them before they scale.
The real AI risks fintech leaders need to manage before they scale
Despite growing confidence in AI, it’s important to be aware that adoption alone doesn’t equal readiness. As the AI in fintech market matures, the most critical risks aren’t limited to edge-case failures or bad predictions; they span the strategic, operational, ethical, and governance layers of how AI is developed and deployed.
In this section, we outline the invisible risks that surface as AI systems move from prototypes to production and especially as they begin to influence credit decisions, fraud detection, customer interactions, and compliance. From model transparency to AI security threats and organizational blind spots, these risks are often overlooked until they trigger reputational damage or regulatory action.
Understanding these categories early will give you the leverage to design more resilient systems, allocate resources more strategically, and make sure artificial intelligence in fintech delivers value without exposing the business to avoidable liabilities.
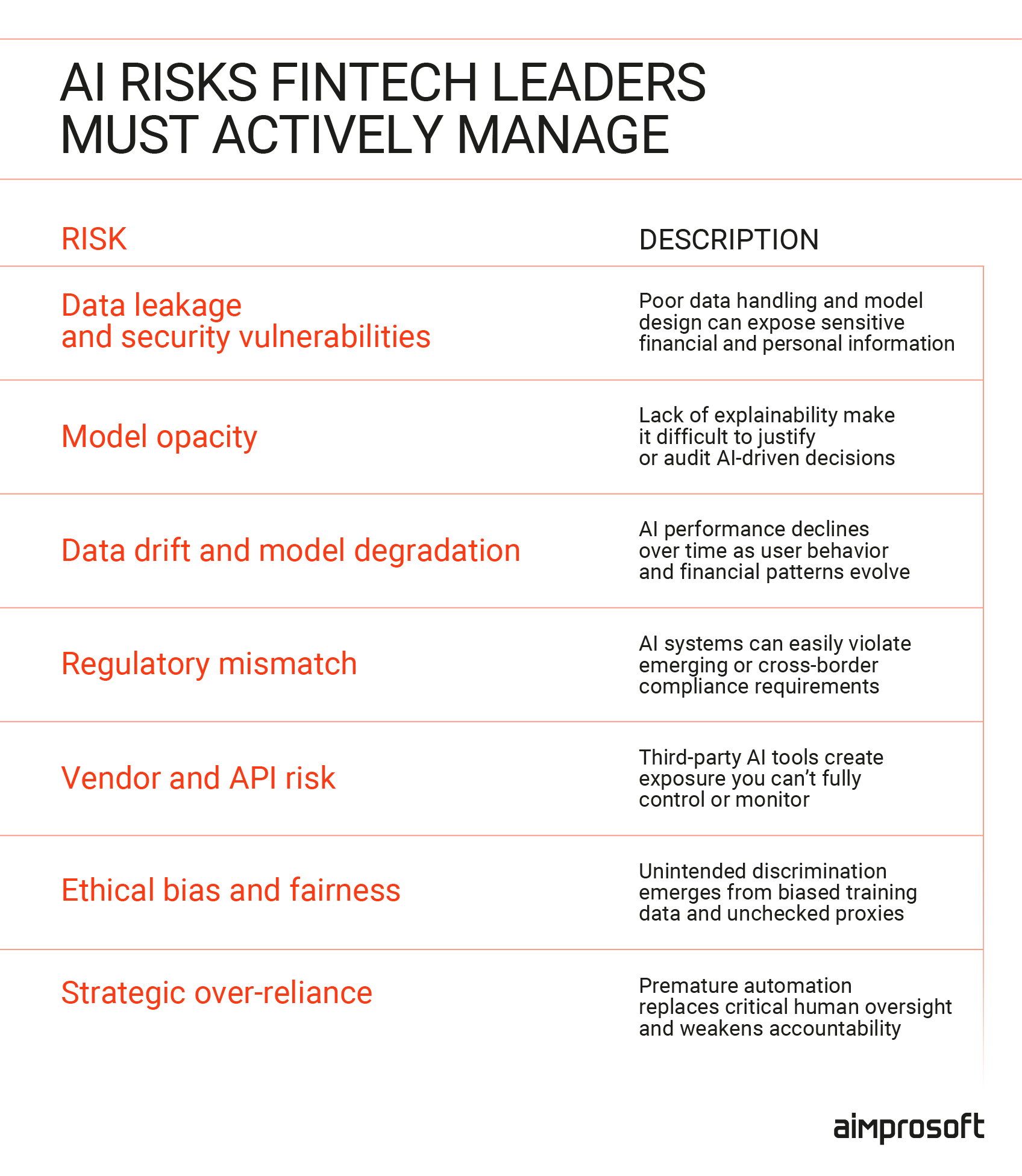
AI risks fintech leaders should know
Data leakage and security vulnerabilities
Artificial intelligence fintech solutions often require ingesting and processing large volumes of sensitive data, from transaction histories and ID documents to behavioral signals and personal communications. While traditional systems follow well-defined data handling protocols, AI in financial technology introduces new pathways for unintended data exposure. This includes leakage through model outputs (in LLMs and generative systems), unsecured data pipelines in training workflows, or inadvertently logging sensitive input data during experimentation.
Fintech companies face an elevated risk because their AI models often interact with third-party APIs, real-time payment data, and personally identifiable information (PII) under strict compliance guidelines (e.g., GDPR, CCPA, PSD2). A single misstep, such as storing training data improperly or exposing internal model prompts, can lead to both regulatory violations and reputational crises. Thus, this risk demands proactive investment in data governance, encryption protocols, role-based access, model sandboxing, and rigorous internal red-teaming to simulate misuse or leakage scenarios before they reach production.
Model opacity
One of the most urgent concerns in AI adoption is explainability. Many AI models, especially ensemble methods or deep learning architectures, produce outputs that are difficult to interpret without dedicated tooling. In a highly regulated environment like fintech, this is more than a technical inconvenience: it becomes a legal and reputational liability. When an applicant is denied a loan or flagged for fraud, you must be able to explain why, and that explanation must stand up to regulatory scrutiny.
Yet, many models are deployed without interpretable layers, audit logs, or documentation of decision paths. This is particularly dangerous when teams use 3rd-party AI solutions for fintech or pre-trained models without fully understanding their inner logic. For fintechs, investing in model interpretability is not optional. It’s a prerequisite for trust, compliance, and long-term viability.
Data drift and model degradation
AI models can degrade over time as user behavior, financial environments, or fraud tactics evolve. This phenomenon, known as data drift, leads to gradual but compounding performance issues: more false positives, poor targeting, or subtle bias. The challenge is that these issues often emerge slowly and invisibly, until they trigger customer complaints or compliance violations.
In production environments, many fintechs lack real-time model monitoring or retraining protocols, especially for models that were initially deployed as MVPs or prototypes. As these models scale, the technical debt grows and eventually compromises product performance, fairness, and decision accuracy. Establishing robust monitoring, drift detection, and retraining pipelines is key to ensuring AI systems remain reliable and legally defensible over time.
Regulatory mismatch
AI systems are moving faster than most regulatory frameworks, but fintech companies don’t get to wait. From the EU’s AI Act to evolving guidelines from the U.S. Federal Reserve and national financial regulators, the landscape is rapidly tightening, especially around explainability, fairness, data governance, and automated decision-making. What complicates the picture is jurisdictional overlap: a model that’s compliant in one market may raise red flags in another.
If you offer cross-border services, this creates a complex compliance burden that many are not yet equipped to manage. Compounding the issue is that many AI fintech features, such as algorithmic scoring or automated onboarding, emerge from product teams with little legal oversight during development. Closing this gap means embedding legal and compliance review directly into AI design and deployment processes, not treating it as an afterthought.
Vendor and API risk
Fintechs rarely build AI systems from scratch. Many rely on third-party vendors for fraud detection, credit scoring, underwriting, or conversational interfaces — a common approach in fintech solutions development with AI, where speed to market often takes priority over full in-house control. While this can accelerate development, it introduces a new category of risk: delegated accountability. When an external model makes a decision that negatively affects a user, whether it’s a loan rejection or a support failure, the liability and reputational damage fall on the fintech, not the vendor.
Unfortunately, many companies integrate these services without full insight into how the models work, how often they’re updated, or whether they meet relevant compliance standards. Even worse, updates to vendor models can happen without notice, silently altering behavior. To truly understand how AI is used in fintech, especially when relying on third-party tools, due diligence must evolve from contract review to technical vetting: asking how models are trained, what data is used, what failsafes exist, and whether outputs can be explained, logged, and contested.
Ethics and fairness
AI systems trained on historical or biased data can inadvertently reinforce inequality, particularly in lending, insurance, and wealth management. What makes this especially dangerous is that biased outcomes may look like rational business logic when driven by proxy variables (e.g., location, spending history) that correlate with protected attributes. The financial consequences for users are real, and the reputational and legal fallout for fintech companies can be severe.
With growing public scrutiny and regulatory attention on algorithmic bias, you need to consider fairness and equity as core model evaluation criteria, not PR add-ons. Techniques like adversarial testing, fairness-aware modeling, and disaggregated performance reporting are becoming essential components of responsible AI practice. At scale, unchecked bias can lead not just to reputational damage but to product bans, litigation, and erosion of public trust.
Strategic over-reliance
As AI systems prove successful in early deployments, many organizations scale them too quickly, automating entire decisions without proper human oversight, contingency planning, or governance structures. This strategic over-reliance creates a dangerous illusion of infallibility. What begins as a tool to support operations becomes the sole decision-maker, whether it’s in fraud flagging, pricing, onboarding, or credit allocation.
When these systems fail (and they will), teams often lack the playbooks or escalation paths to intervene effectively. You must resist the urge to automate prematurely and instead focus on phased deployment, human-in-the-loop decision systems, and the development of internal AI fluency across legal, product, and executive functions. AI can enhance decision-making, but when used uncritically, it can also obscure flawed assumptions behind a veneer of objectivity.
Summary: what you should watch for as a fintech leader
If you’re skimming for key takeaways, here’s a quick breakdown of the core AI risk categories and why they matter:
- Data leakage and security vulnerabilities expose sensitive financial and identity data through unmonitored AI pipelines, training logs, or model outputs.
- Model opacity undermines accountability when decisions can’t be explained — a major issue in regulated domains like lending and fraud detection.
- Data drift and model degradation quietly erode performance over time, leading to inaccurate outcomes and compliance exposure.
- Regulatory mismatch occurs when AI systems outpace evolving legal standards, especially across international markets.
- Vendor and API risk introduces uncertainty when third-party models make decisions your users hold you accountable for.
- Ethical bias and fairness failures can result in systemic discrimination, reputational damage, and long-tail legal consequences.
- Strategic over-reliance on AI without proper oversight can lead to automation replacing critical thinking and human judgment too soon.
Each of these fintech industry challenges requires proactive attention not just from data scientists but from product owners, legal teams, and executives. In the next section, we’ll break down how to build a practical, cross-functional AI governance framework and AI management strategy tailored to fintech realities.
Is your company ready to manage AI risks? A strategic readiness framework
Understanding AI risks is only half the equation; the other half is knowing whether your organization is actually prepared to handle them. At this stage, most fintechs don’t lack awareness. They lack a cross-functional lens to assess how fragmented or mature their AI risk posture really is. As the disadvantages of AI in finance become more evident in real-world deployments, AI financial risk management is becoming a critical capability, one that requires more than surface-level understanding.
Below is a structured framework to help you interrogate your company’s readiness across five critical domains, blending technical, operational, and compliance criteria. This isn’t a best-practice list; it’s a diagnostic tool for identifying risk exposure before it scales.
1. Ownership & accountability
- Do you have named owners for every AI model in production, including their retraining schedules, data sources, and business use cases?
- Is there an escalation path when a model underperforms, violates policy, or produces unexpected outcomes?
- Are compliance and legal teams involved before an AI product or feature is shipped?
Why this matters: Many AI failures don’t happen because the model was wrong. They happen because no one was responsible for watching it closely after launch.
2. Visibility & documentation
- Can your team produce an audit trail for each AI-driven decision that impacts a user, especially in lending, fraud, or customer service?
- Are your third-party AI fintech tools documented with model access logs, version histories, and change notifications?
- Do you maintain model cards or similar artifacts describing model purpose, limitations, and performance?
Why this matters: Regulators increasingly expect explainability-by-default. Lack of documentation is one of the fastest paths to failed audits or paused products.
3. Data governance & lineage
- Do you track where your training and inference data originates and whether it includes PII, sensitive attributes, or outdated information?
- Do you validate whether third-party data used for AI is compliant with local and international regulations (e.g., GDPR, CCPA, AI Act)?
- Is there a formal process for updating datasets and versioning them as models evolve?
Why this matters: Poor data lineage is a hidden liability. It affects fairness, compliance, and repeatability, especially during investigations or regulatory reviews.
4. Monitoring & drift detection
- Are all production models monitored for performance, fairness, and accuracy, in real time or near-real time?
- Can you detect data or concept drift automatically, and do you have thresholds that trigger retraining or review?
- Are these monitoring tools accessible to product and compliance teams, not just engineers?
Why this matters: Drift is one of the most common silent AI failures. If you can’t catch it early, it will compound until customer experience and compliance are both compromised.
5. Third-party and vendor model risk
- Do you evaluate external AI tools beyond just functionality, including how models are trained, updated, and monitored?
- Is there a review process when third-party models change or retrain, especially if they directly influence end-user experience or compliance?
- Do you log and monitor outputs from vendor models integrated into your platform?
Why this matters: When third-party AI tools go wrong, your brand is still the one customers blame and regulators pursue.
Let’s walk through it together. Get in touch to discuss how we can help you evaluate and strengthen your AI risk posture.
CONTACT USAI adoption in fintech doesn’t just require smart engineers and ambitious roadmaps. It demands organizational clarity, operational discipline, and ongoing ownership. This readiness framework isn’t about slowing innovation. It’s about making sure the innovation you deploy is resilient, auditable, and aligned with the level of risk your company is willing (or allowed) to carry.
Turning insight into action. How to mitigate AI risks before they become failures
Understanding the risks of AI is essential, but preventing them is where leadership earns its edge. In this section, we provide actionable strategies to manage the invisible risks outlined earlier, not by halting innovation, but by embedding control, resilience, and transparency into AI systems from day one.
Each set of recommendations below is tailored to the specific nature of the risk and based on practices we’ve seen work in high-stakes fintech environments.
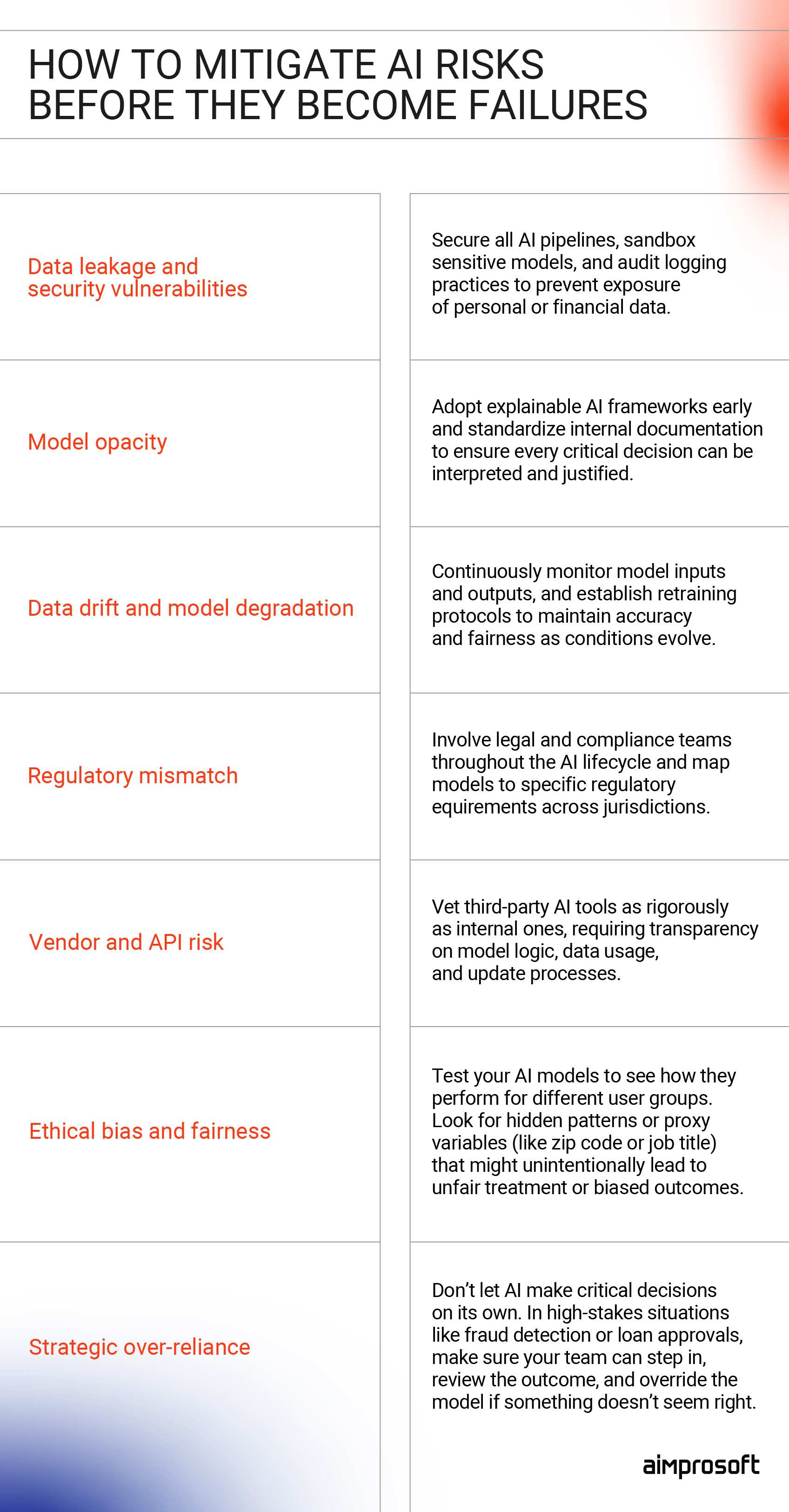
How to mitigate AI risks
Model opacity: ensure explainability before the audit
As AI becomes embedded into decision flows, especially in lending, fraud, and onboarding, unexplained outputs are no longer acceptable. You must prioritize explainability not as an add-on, but as part of the model lifecycle.
Mitigation tips:
- Use model-agnostic explainability tools (e.g., SHAP, LIME) during both training and inference phases.
- Set internal explainability standards based on the risk profile of each model.
- Maintain detailed model cards describing logic, assumptions, limitations, and intended use cases.
- Require third-party vendors to provide transparency artifacts or interpretable API layers.
- Train cross-functional teams to understand and communicate AI-driven outcomes to regulators or end users.
Data drift and model degradation: monitor performance as a moving target
Even well-trained models degrade when the data ecosystem shifts. Behavior patterns, fraud tactics, and user cohorts change, and models must evolve with them.
Mitigation tips:
- Implement automated drift detection tools to monitor input and output distribution shifts.
- Establish retraining schedules based on model usage frequency, business impact, and detected drift.
- Tie model performance to business metrics (e.g., approval rates, false positives), not just statistical accuracy.
- Log production data for regular validation without exposing sensitive customer information.
- Use shadow deployments to test retrained models in parallel before replacing live ones.
Regulatory mismatch: align AI models with the legal landscape
The gap between model development and legal review is where most compliance failures begin. Instead of treating AI regulation as a blocker, integrate it as part of the build process.
Mitigation tips:
- Create internal review checklists that tie AI features to regulatory obligations (e.g., explainability, fairness, auditability).
- Involve compliance and legal stakeholders at the scoping stage, not post-launch.
- Maintain jurisdictional intelligence, track evolving standards like the EU AI Act, U.S. Fed guidance, and regional AML rules.
- Document user-impacting decisions made by AI systems and log reasoning where required.
- Review models regularly against updated interpretations of fairness, discrimination, and automated decision-making rules.
Vendor and API risk: demand transparency and control from third parties
Third-party models often power critical functions, such as fraud detection, scoring, and even LLM-based chat. But delegating functionality doesn’t mean delegating accountability.
Mitigation tips:
- Require vendors to disclose model architecture, data sources, retraining frequency, and update notifications.
- Set SLAs that include not just uptime, but fairness, auditability, and explainability standards.
- Route vendor outputs through internal validation or override layers before exposing them to users.
- Log all decisions made by third-party models, especially if they impact user rights or access.
- Revalidate vendor models when switching user bases, markets, or regulatory environments.
Ethical bias and fairness: test for harm before it happens
Bias isn’t always intentional. It often emerges from data proxies, historical trends, or imbalanced training sets. Addressing this risk requires more than disclaimers; it takes proactive testing and monitoring.
Mitigation tips:
- Evaluate model performance across different user segments, even when protected attributes are not explicitly included.
- Use synthetic or adversarial data to test how edge cases and minority groups are treated.
- Incorporate fairness metrics into validation (e.g., equal opportunity, disparate impact).
- Build internal escalation paths for teams to challenge biased outputs or retrain faulty models.
- Red-flag proxy variables (e.g., zip codes, employment history) that may encode systemic bias.
Strategic over-reliance: keep human oversight in the loop
When teams begin trusting AI too quickly or too deeply, critical thinking erodes. Strategic over-reliance can blind organizations to nuance, exception handling, or ethical red flags.
Mitigation tips:
- Implement tiered automation, allow AI to suggest, but not always decide, in early phases.
- Establish override systems and give frontline teams the authority to flag or reject model outputs.
- Run regular AI failure drills to simulate what happens when a model makes the wrong call and test how your teams respond.
- Ensure product owners and operational leaders understand model limitations, not just performance stats.
- Maintain a “slow path” for sensitive decisions like fraud bans, loan rejections, or escalated support cases.
Data leakage and security vulnerabilities: treat AI pipelines as attack surfaces
AI systems expand the threat surface. From training datasets to model outputs, every step of the pipeline must be secured, especially in a data-sensitive sector like fintech.
Mitigation tips:
- Classify AI training and inference data under the same protections as production user data.
- Enforce access controls on datasets, model artifacts, and inference endpoints, especially for internal LLMs or generative models.
- Audit log inputs/outputs of models handling sensitive data and redact where necessary.
- Regularly test models for PII leakage, prompt injection, or exposure through API access.
- Deploy new models in sandboxed environments before production rollout, using red-teaming to identify vulnerabilities.
Our team can support you with secure, scalable AI integration tailored to your business.
CONTACT USWrapping up
The risks and threats of AI in fintech aren’t edge cases; they’re systemic, evolving, and deeply tied to how your organization builds, scales, and governs its technology. As AI moves from isolated use cases into the heart of lending, fraud, and customer operations, the cost of reactive thinking grows exponentially.
The good news? These risks are manageable, not by slowing innovation, but by embedding the right controls, ownership, and visibility from the start. Fintech leaders who treat AI governance as a core capability, not a compliance checkbox, will move faster, scale smarter, and build platforms that users and regulators can trust.
If you’re ready to move from cautious experimentation to confident execution, get in touch with our team. With our fintech AI development services, you will get a scalable AI fintech solution tailored to your fintech business and securely integrated into your business operations.
FAQ
How do we balance speed of AI deployment with the need for risk controls?
It’s a common misconception that fintech risk and compliance management slows innovation. In reality, the most advanced fintech companies embed lightweight guardrails early, not as roadblocks, but as scalable enablers. By defining risk thresholds, establishing model ownership, and using modular review checklists from the start, teams can move fast without losing control. The key is to align risk checkpoints with existing dev or product lifecycles, not add parallel bureaucratic processes that delay releases.
Do we need an internal AI governance team, or can AI risk management be performed cross-functionally?
Both models can work but what’s essential is clear accountability. You don’t necessarily need to establish a dedicated AI governance office, but you do need clearly defined roles across data science, compliance, product, and legal. A centralized AI risk management framework can guide distributed teams: defining what “responsible AI” means in your company, when employee review is required, and what red flags should trigger escalation.
What kind of documentation is required to meet requirements of auditors or regulators?
Regulatory expectations vary by region, but increasingly, authorities expect traceability around how models are developed, validated, monitored, and updated. Key documentation includes:
- Model cards that describe purpose, logic, limitations, and performance metrics;
- Data lineage logs showing where training data comes from and how it’s handled;
- Decision audit trails for high-impact outcomes (e.g., loan rejections, fraud flags);
- Monitoring reports that show how performance, fairness, and drift are tracked over time.
Proactive documentation doesn’t just satisfy audits, it makes your AI stack more maintainable and defensible long-term.