The State of Agentic AI in Business: Meet the Do-It-For-Me Era
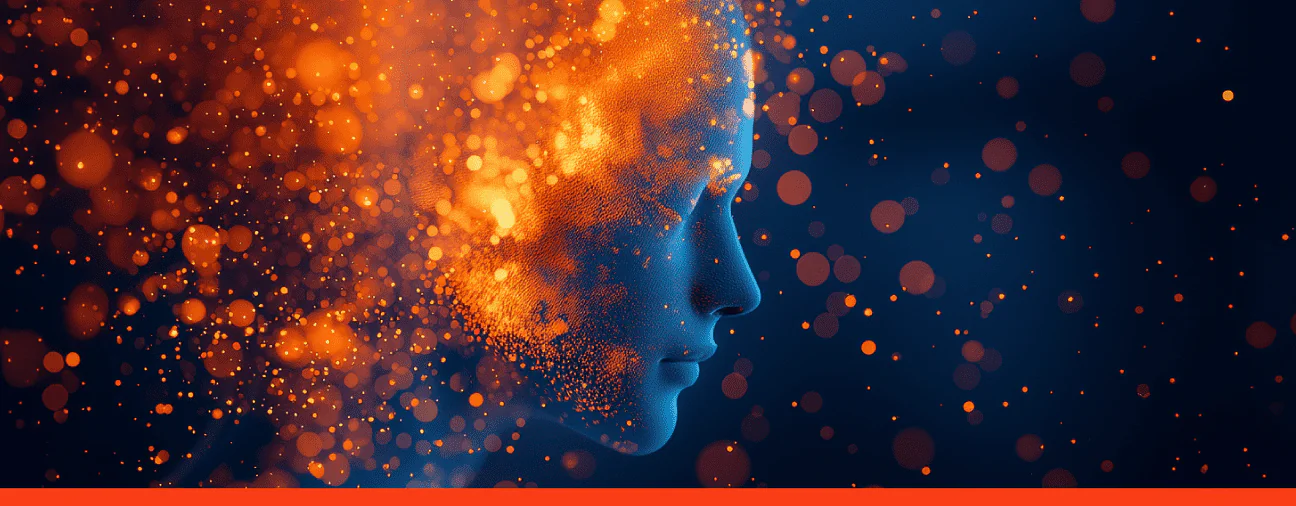
Artificial intelligence is literally everywhere. It suggests your playlist for the road, writes follow-up emails, and even detects cancer on X-rays. But there’s one catch—it still needs you to tell it what to do. Every action starts with an instruction.
And while many see Gen AI as the peak of automation, this perception is about to change as we enter the AI agentic era.
Agentic AI is a new wave of autonomous systems that don’t just assist but act. Instead of waiting for instructions, they anticipate needs, execute tasks, and drive outcomes with minimal human intervention.
Agents transform the entire AI concept, maturing it from a passive tool into an autonomous decision-maker capable of delivering real business impact. Gartner predicts that by 2028, 33% of enterprise software will have agentic AI built in.
The question is: Will your business be among them?
In this article, we’ll break down agentic AI definition, explore real-world agentic AI examples, and dive into how businesses can harness AI agents for automation and decision-making.
Everything you wanted to know—right here in one place.
What is Agentic AI?
Agentic AI is an autonomous or semi-autonomous system that uses AI techniques to understand its environment, make decisions, act, and work toward goals in both digital and real-world contexts. Unlike traditional AI, which follows strict instructions, agentic AI can adapt and respond to new information on its own.
Since agentic AI can work independently, it’s often mistaken for other AI technologies like large language models (LLMs), robotic process automation (RPA), and chatbots. While these technologies can be part of an agentic AI system, they don’t automatically make AI agentic. See the explanations below:
- Large language models (LLMs) are AI systems that understand, generate, and process human language. Agentic AI can leverage them to interpret prompts and provide textual feedback, but not all LLMs are agentic. For instance, ChatGPT uses LLMs, and if you ask it to build a website, it would suggest a step-by-step guide or generate code snippets—but it wouldn’t execute the task itself. But agentic AI would choose the right tools, write and deploy the code, and continuously refine the website based on real-time feedback.
- Robotic process automation (RPA) automates repetitive, rule-based tasks. But it lacks adaptability. An RPA bot that processes invoices can’t handle unexpected problems like missing data. It simply stops. Agentic AI, on the other hand, can analyze problems, adapt its approach, and even attempt to resolve them independently. For example, it might retrieve missing information or request clarification.
- Conversational assistants like chatbots are designed to interact with users via text or speech. They can answer questions, assist with customer support, or give recommendations, but they rely on predefined scripts or models. For example, if you ask a chatbot to schedule a meeting, it might send you a link to a scheduling tool. Agentic AI, in contrast, goes beyond assistance. It can autonomously book meetings, coordinate schedules, and handle unforeseen issues, requiring minimal user intervention.
As you can see, agentic AI can use all these technologies to reach its goals, but none of them alone define what agentic AI is.
Key Aspects of Agentic AI
To ensure we’re on the same page regarding the meaning of agentic AI, we’ve gathered some key points that help distinguish it from other AI systems.
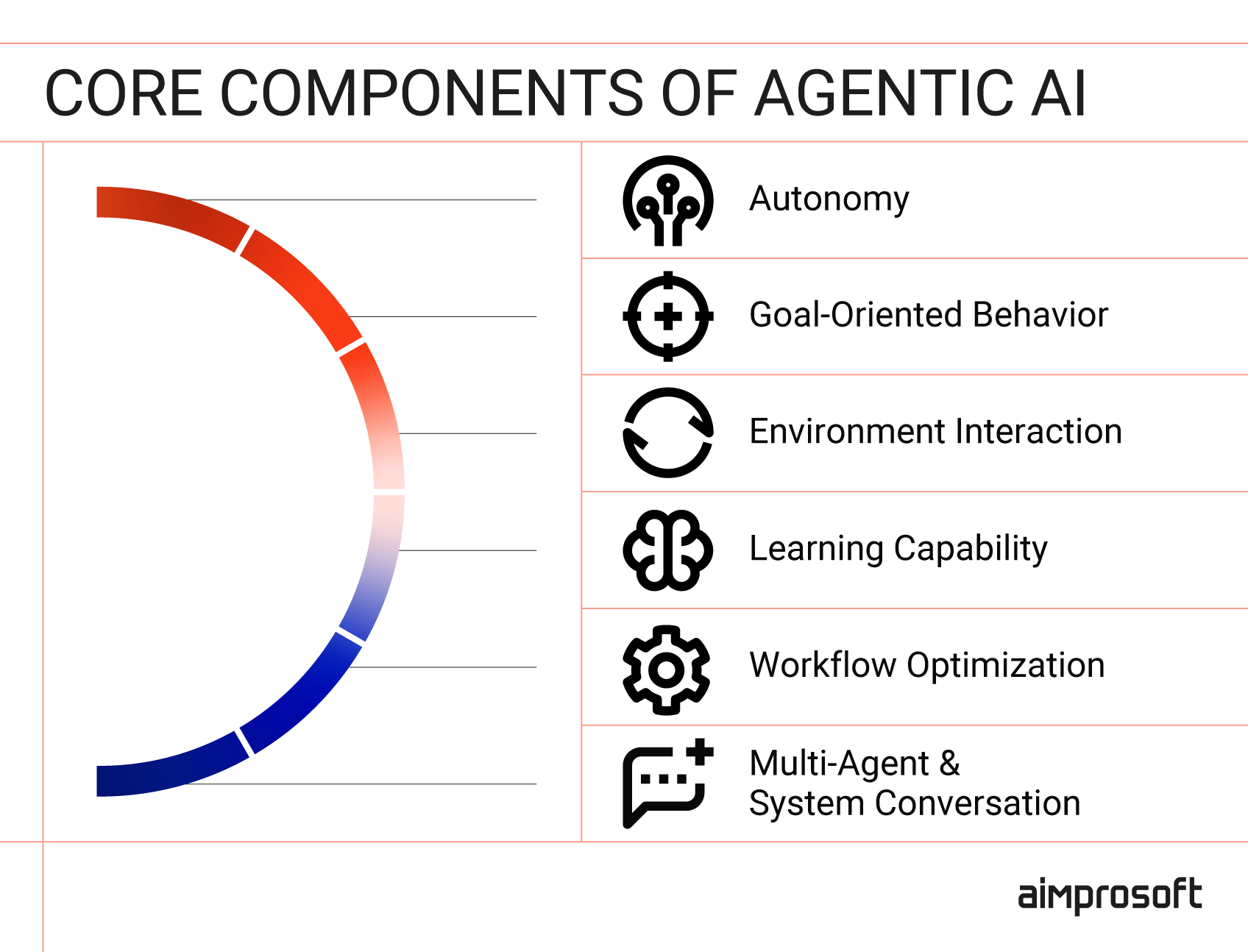
- Autonomy—Unlike traditional AI, agentic AI systems can operate independently, making decisions, learning, and providing additional inputs (that may not be mentioned in the original prompt).
- Goal-oriented behavior—AI agents don’t simply answer questions but help the user achieve their goals (a self-driving car’s goal is not just to move but to safely and efficiently reach its destination).
- Environmental interaction— Agentic AI constantly collects information from its environment, understands changes, and adjusts in real-time to make better decisions and complete tasks efficiently.
- Learning capability—Many agentic AI models use machine learning or reinforcement learning to learn from past experiences, recognize patterns, and refine their behavior over time.
- Workflow optimization—By combining language perception with reasoning and decision-making capabilities, agentic AI can streamline how things get done. For instance, an AI-powered customer service system can analyze user’s inquiries, identify issues, and route them to the right department or agent.
- Multi-agent and system conversation— Agentic AI isn’t isolated—it interacts with other agents, tools, and systems to complete tasks. Meaning you can have multi-agent AI that works like a real team.
Now that we’ve covered what is an agentic AI and its core elements, let’s peek under the hood to see how it works.
Agentic AI Workflow
While different systems may have unique architectures, there’s a core four-step agentic AI framework: Perception, Reasoning, Acting, and Learning.
This structure forms the backbone of agentic workflows in AI, allowing it to process information, make decisions, act, and refine its strategies over time. Now, let’s delve into details.
1. Perception
Think of this as the AI’s senses. This module gathers data from various sources like APIs, databases, and sensors. It then uses AI techniques like computer vision and NLP to understand what it’s seeing and hearing, turning raw data into meaningful information (steps needed to complete the task).
2. Reasoning
This is where the AI starts to think. It sets goals, plans its approach, and optimizes decisions in real-time. It also assesses risks and adapts strategies to ensure the best possible outcome.
3. Acting
As the name suggests, this is where things get done. The AI executes its decisions by interacting with external tools, APIs, and systems. It tracks progress, adapts its actions if needed, and ensures the job is completed efficiently.
4. Learning
Like humans, agentic AI learns from its successes and failures. Using techniques like reinforcement learning, it analyzes past performance and adapts its strategies for continuous improvement.
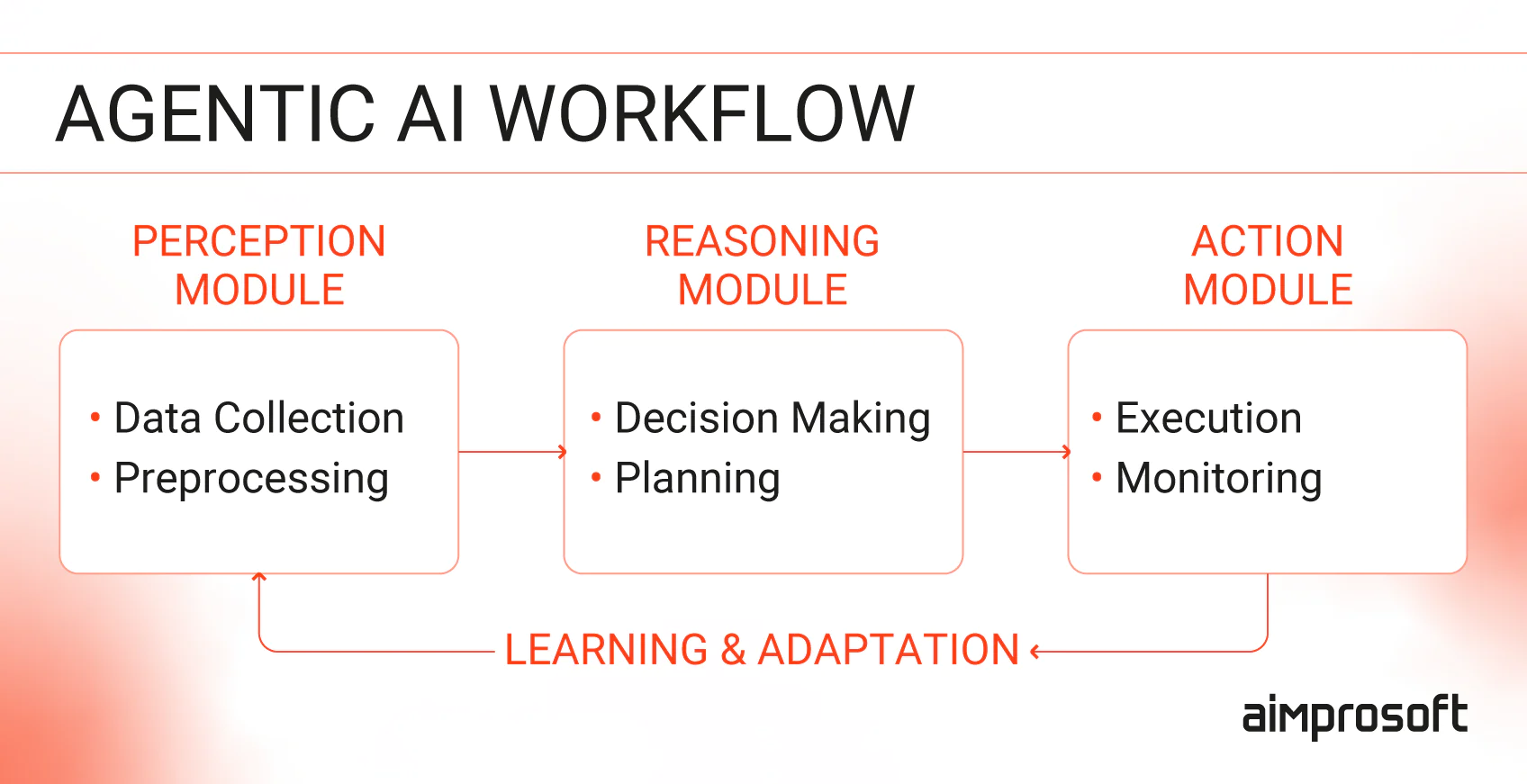
Thanks to this combination of steps, agentic AI can optimize processes, anticipate changes, and adapt on its own, instead of waiting for further instructions.
Understanding Agentic AI Patterns
The Perception → Reasoning → Acting → Learning workflow is the backbone of agentic AI. But while this core process stays the same, how it’s built and fine-tuned can vary.
Depending on the use case, developers can choose different frameworks and customize components—also known as patterns—to optimize AI performance. In agentic AI, these patterns fall into two key categories: functional and operational.
Functional Patterns: Define how AI agents think, interact, and make decisions (e.g., reasoning, memory, action execution).
Operational Patterns: Focus on governance, evaluation, and security, ensuring AI agents function reliably and ethically.
Functional Patterns
How AI Agents Are Organized
Agentic AI architecture comes in many forms, ranging from single, independent agents to complex networks of collaborating agents. How they’re organized makes a huge difference in how well they perform. Here’s an example of how AI agents are set up:
- Solo agent: A single AI agent works independently to complete a task from start to finish.
- Agent roles: AI agents specialize in specific tasks within a larger system, like a team where everyone has a defined role.
- Agent-to-agent handoff: One AI agent starts a task and passes it to another to keep things running smoothly.
- Multi-agent modularity: In this setup, agents are organized into a network with well-defined responsibilities, allowing flexibility and scalability across complex workflows.
How AI Plans and Solves Problems
The best part of agentic AI is that it’s not just about following instructions—but planning and adapting. Different strategies help AI handle uncertainty, work through complex tasks, and balance automation with human input. Here are some of them:
- Prescribed plan: AI follows a fixed, pre-defined set of rules and procedures.
- MHQA (Multi-Hop Question Answering): AI pulls information from multiple sources before answering, ensuring a more accurate response.
- Orchestrated agents: This approach involves multiple AI agents working together in a coordinated manner, often with a hierarchical structure.
- Dynamic plan generation: With this strategy, AI systems adapt their plans in real time based on new information, unexpected changes, or changing objectives.
- Collaborating agents: Here, multiple AI agents work together dynamically to solve a problem, often sharing information and coordinating their actions.
- Human-in-the-loop: AI consults a human for critical decisions to ensure accuracy and alignment with real-world needs.
How AI Thinks and Learns
Agentic AI doesn’t just act; it learns from its actions. Due to these cognitive patterns, agentic AI processes information, adapts, and improves its performance. Here are a few examples of how agentic AI can learn and improve:
- ReAct (reason + act): This pattern allows AI to analyze the situation, consider options, and then act (step-by-step).
- Chain of thought: AI explains its reasoning process before making decisions, improving transparency. It also helps identify potential biases or errors.
- Structured response: AI formats its outputs in structured data, like tables, JSON files, or text, to ensure clear and concise communication.
- Reflexion: AI analyzes its past actions and identifies areas for improvement.
- Retry limits: This strategy prevents the AI from getting stuck in endless loops. By limiting the number of attempts, the AI can avoid wasting time and resources on unproductive tasks.
How AI Performs Tasks
True autonomy for AI lies in its ability to take action in the real world. To do so, agentic AI systems utilize various mechanisms, such as using APIs, generating code, and integrating with other tools to complete tasks. Here are a few examples of how they work:
- Function calling: AI calls APIs or external services to complete tasks (e.g., fetching data, performing calculations, triggering actions).
- Generated code execution: This pattern allows agentic AI to write, modify, and run code to achieve its goals.
- API tool use: AI integrates with third-party platforms like CRM systems, databases, or automation tools to manage workflows and synchronize data.
How AI Stores and Uses Information
For agentic AI to truly learn and grow, it needs a robust memory system. These patterns define how AI agents manage and use knowledge effectively:
- Memory longevity: It allows agentic AI to learn from successes and failures, improving its decision-making over time.
- Memory scope: AI determines what to remember and what to forget, balancing efficiency with contextual awareness. This prevents memory overload and ensures efficient resource utilization.
- RAG (Retrieval-Augmented Generation) empowers AI to access and use external knowledge bases, such as databases or the Internet, to supplement its internal knowledge instead of relying solely on pre-trained data.
Operational Patterns
How AI Is Monitored and Assessed
AI needs oversight to make sure it’s safe, reliable, and actually doing what it’s supposed to. These evaluation methods help measure AI performance and identify potential issues:
- User-in-the-loop involves humans reviewing and guiding AI decisions. This ensures that the AI’s actions align with human values and expectations.
- LLM-as-a-judge relies on other AI models to assess the quality and relevance of generated outputs.
- Deterministic (Code-Based) evaluation: AI follows a set of pre-defined rules to measure the AI’s performance.
- Interaction logging: AI keeps records of decisions, actions, and interactions. This provides valuable data for analyzing performance, identifying potential biases, and debugging issues.
- Red teaming: AI is tested in difficult or unexpected situations to identify weaknesses and vulnerabilities.
Ensuring AI Operates Securely
Security is paramount for agentic AI systems. These patterns help you ensure safe and responsible AI operations by addressing security and ethical considerations:
- Identity token propagation ensures that AI systems can securely manage user identities and permissions. This prevents unauthorized access and ensures that legitimate users perform AI actions.
- LLM guardrails are implemented to prevent AI systems from generating harmful, biased, or unethical outputs. These safeguards ensure that AI operates within ethical boundaries and respects human values.
These patterns are not strict rules. They serve as best practices to help developers build smarter, safer, and more efficient agentic AI solutions. Tech experts can choose relevant practices to develop an agentic AI that meets specific business needs using popular agentic AI tools. Alternatively, you can select ready-made solutions that are available through agentic AI platforms.
Agentic AI Tools and Platforms
Although agentic AI applications are designed to work autonomously, they must first be developed. To build these systems, businesses and developers rely on two core components:
- Agentic AI tools: These include specialized frameworks, libraries, and SDKs that enable developers to build autonomous AI agents. Such tools are building blocks for creating AI systems that can understand context, plan actions, and learn from outcomes.
- Agentic AI platforms: Ready-to-use platforms that offer secure, scalable environments for running autonomous AI agents. They make it easy for agentic AI companies to launch solutions by providing built-in agents, seamless integration, and powerful monitoring tools.
Below, we’ve made an overview of key tools and platforms that can be used to develop your agentic AI projects.
LangGraph
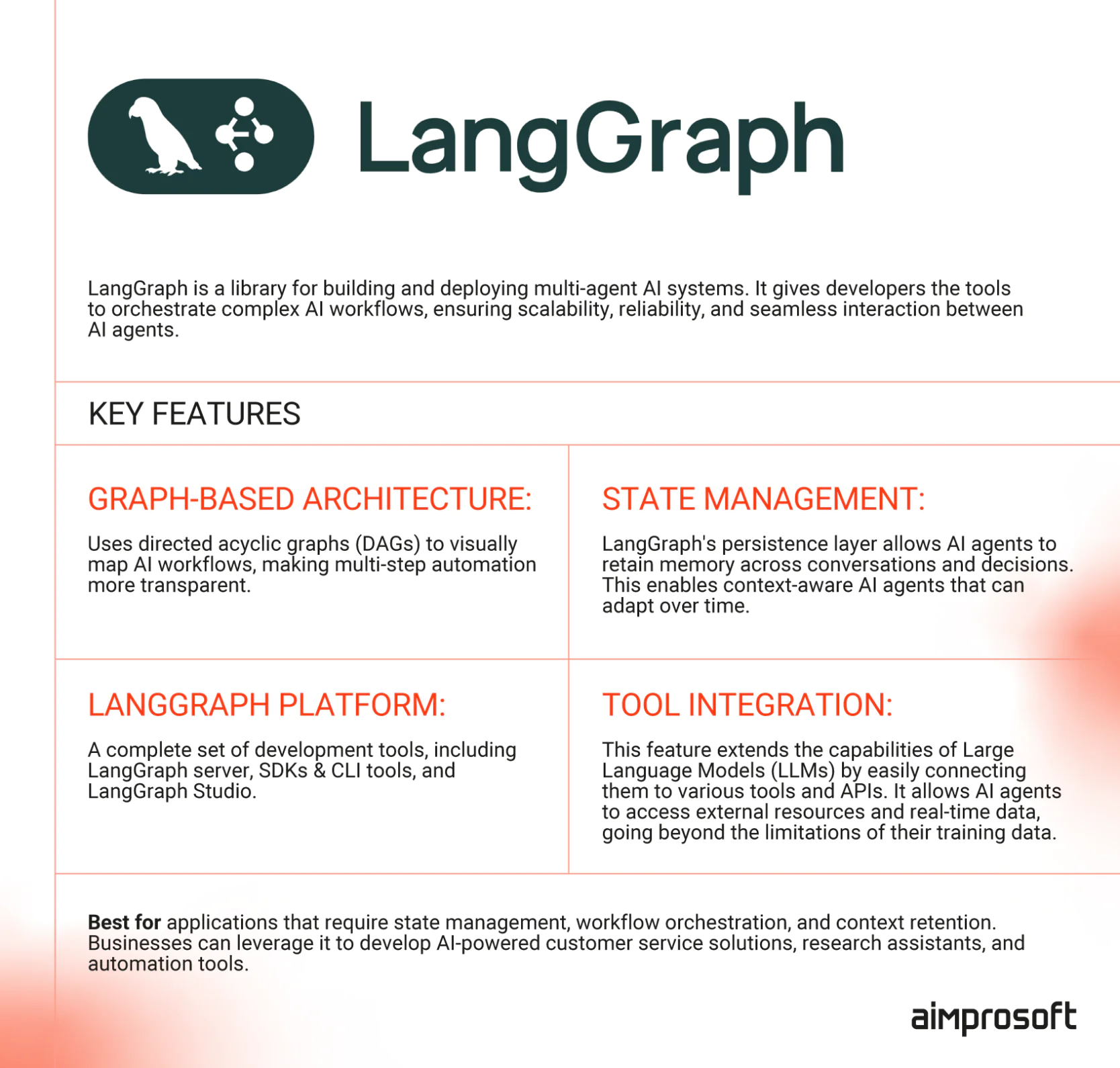
LangGraph is a developer-friendly library for building and deploying multi-agent AI systems with complex workflows and persistent memory. It enables efficient collaboration between AI agents, making it ideal for applications that require state management and adaptive decision-making.
If you need an agentic AI system that retains context, structures workflows, and adapts dynamically, LangGraph is the way to go.
AutoGen
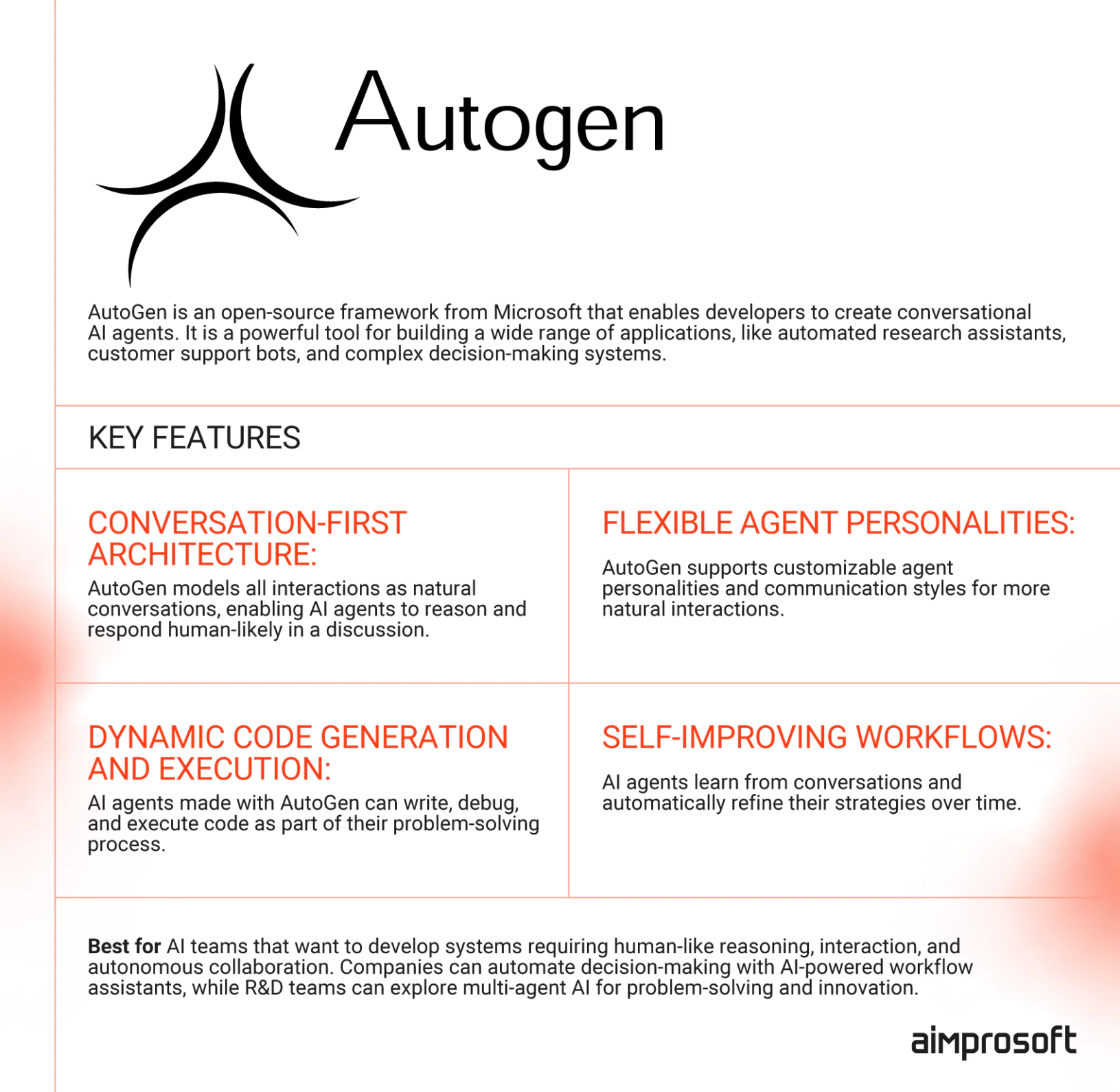
AutoGen is an open-source framework for developing AI systems where multiple agents work together to independently solve tasks. It enables AI agents to communicate, call functions, write and run code, and complete tasks on their own. This setup creates an AI agentic workflow, making it great for AI-powered assistants and decision-making systems.
If you want AI that collaborates naturally—like human teams—AutoGen is built for fluid multi-agent interactions.
CrewAI
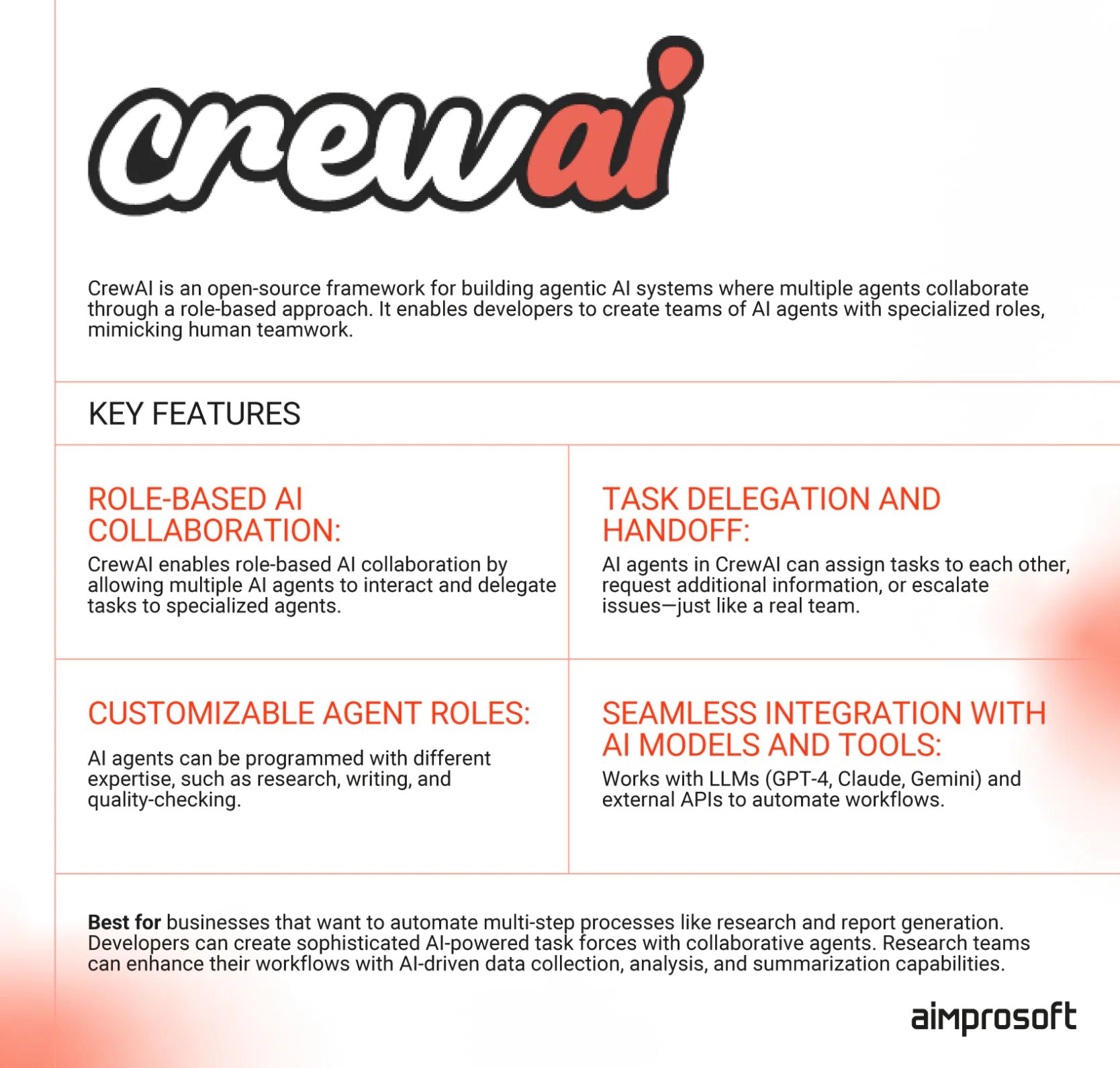
CrewAI is a multi-agent AI framework that organizes AI agents into specialized roles, allowing them to work together like a real-world team. It is designed for AI systems that require structured collaboration and task delegation. The best use cases are research, report generation, and content curation.
For teams developing structured, multi-agent AI systems, CrewAI provides the best agentic AI framework for role-based collaboration.
Krista AI
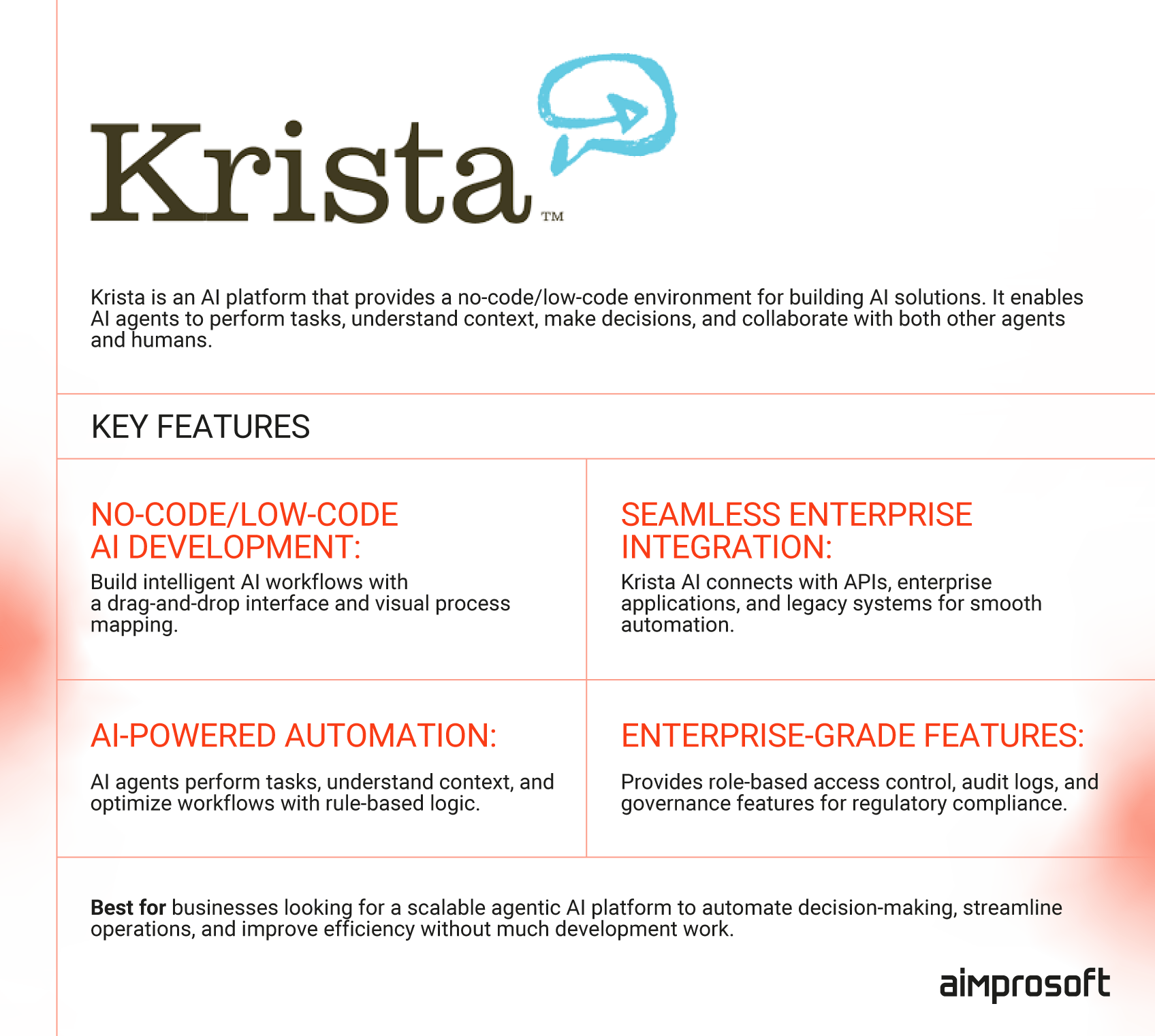
Krista AI makes AI adoption seamless with its no-code/low-code platform, enabling businesses to automate workflows, manage AI-driven decision-making, and integrate AI agents effortlessly. Designed for accessibility, it allows organizations to deploy intelligent automation at scale without technical complexity.
Want agentic AI without writing code? Krista AI is a business-friendly platform that enables effortless automation with no-code AI agents.
UiPath
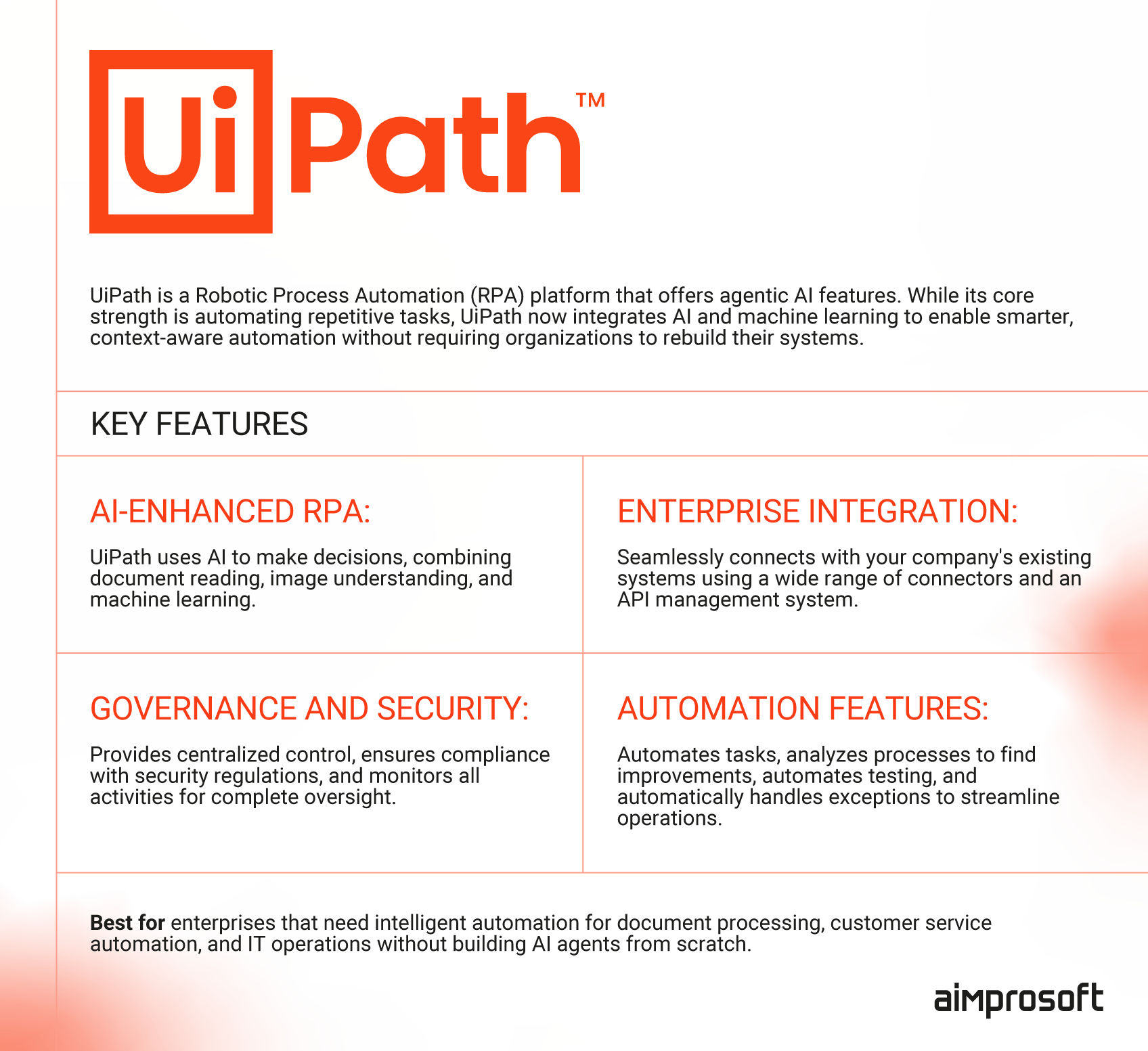
UiPath is an AI-driven RPA platform that blends traditional automation with AI-powered decision-making. Unlike frameworks like LangGraph and CrewAI, which focus on AI agent development, UiPath provides a structured, no-code environment for streamlining business processes. It enables users to build agentic AI workflows that analyze data, set goals, and operate with minimal human oversight—without requiring coding expertise.
Already using RPA but need smarter automation? UiPath blends AI with traditional process automation to scale agentic AI for your business.
Agentic AI Use Cases
Agentic AI has been proven to work well not only on paper but also in practice. Many worldwide companies are already applying this technology to their work and reaping the fruits of AI’s agency.
For instance, Juna.AI uses AI agents to run virtual factors to enhance production speed and reduce energy consumption. And this is just one example, as agentic AI’s potential in manufacturing is enormous. Agentic AI could be used to control and enhance the flow of production lines, provide suggestions for improving product design, and monitor the performance of machines using IoT sensors.
Agentic AI also found its place in the healthcare domain, with Hippocratic AI leading the way. Their marketplace of AI-powered agents for low-risk, non-diagnostic, patient-facing services is making strides. Agentic AI solutions can create patient treatment plans based on discoveries and test results, follow up with patients about their treatment, and even monitor vital signs on behalf of doctors.
Agentic AI solutions also make sales teams more efficient and productive. For instance, Salesforce already implements agentic AI tools that assist sales teams in interpreting customer messages, recommending follow-up actions, or scheduling meetings.
Summing Up
In the face of rapidly advancing agentic AI, businesses must act swiftly to avoid being left behind. You can either conquer AI by choosing the tools and platforms best suited to your needs, or partner with experts like Aimprosoft to handle heavy lifting.
At Aimprosoft, we provide AI consulting services to help you define strategies, identify automation opportunities, and bridge the gap between AI teams and stakeholders. We assist with your biggest challenges, including performance monitoring and compliance, while our AI-native app prototyping enables quick pilots with no-code/low-code tools.
Just reach out to us—we’ll help you build an AI integration roadmap and connect you with the right tools and experts to make it happen.