Machine Learning in Healthcare Projects: From Not-For-Everyone Treatment To Mass-market
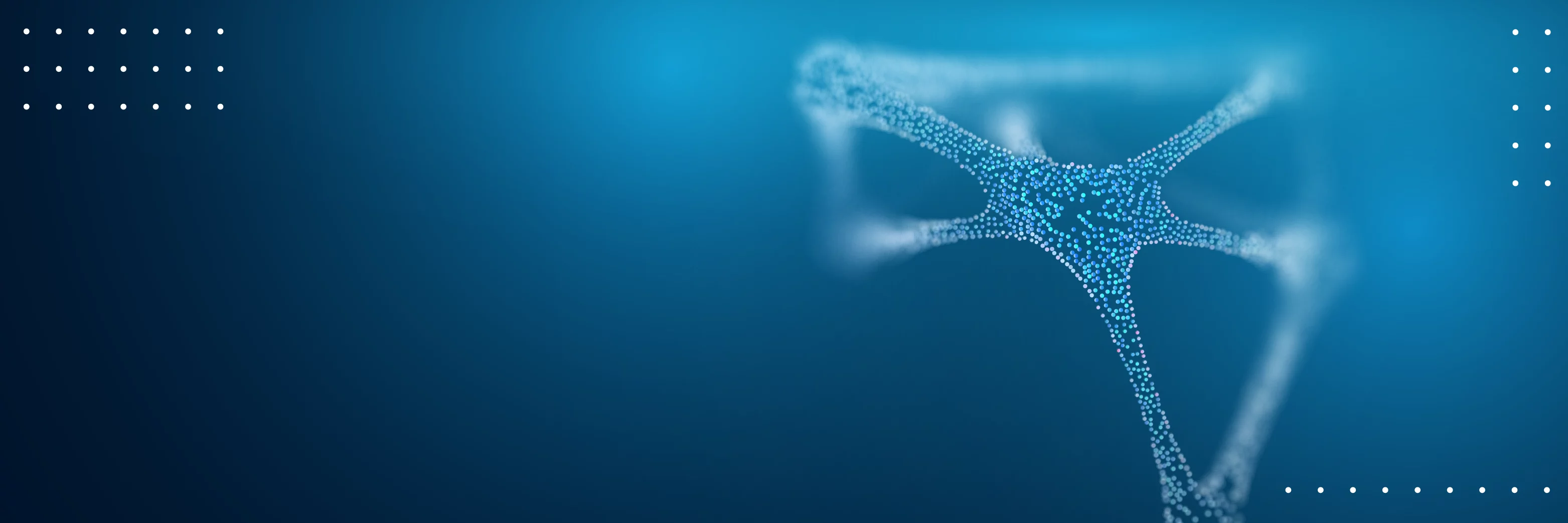
Key takeaways
- We’ll go through the main factors that trigger ML evolution in healthcare and why trillions of dollars may be the case.
- Following the story, you are able to discover notorious machine learning applications in healthcare that may spark a thought for new opportunities.
- You’ll get acquainted with the ethical and regulatory dimensions of AI/ML usage when you’re going to make a medical app for the particular purposes of your business.
- Also, learn more about Aimprosoft’s capabilities in the data science area.
Machine learning in healthcare is gradually taking over the world: until recently, it seemed unimaginable, but now it is rapidly becoming popular.
Since 1972 MYCIN, an early AI-based expert system program backed by Stanford University, has become operational to treat blood infections. It’s gone almost four decades till the first commercial applications entered the market with revolutionary solutions that transform the industry and save people’s lives.
According to the recent study findings, there are 690 AI-powered startups actively developing healthcare solutions globally. 3D image analysis, disease identification, administrative work enhancement, robotic surgery, intelligence biosensors, and many other applications inculcate great optimism about the directions of healthcare use cases for machine learning.
Let’s see how a constant expansion of the range of challenges to be solved with AI/ML can affect the quality of healthcare delivery opening new business opportunities.
Evolution and perspectives of machine learning in healthcare
The simulation of intelligence by machines, which is artificial intelligence, boils down to a system that seems smart and the way this system becomes smart, which is machine learning. In a nutshell, it can take massive amounts of healthcare data and improve ways of processing and understanding data under the simple rules of the “if-then-else” variety.
ML algorithms implement mathematical models to run supervised and unsupervised machine learning. Supervised learning is a simple ML technique (often in Python, e.g., for classification) aimed to predict outcomes from new data. Unsupervised learning is highly computationally complex and requires robust tools to deal with large amounts of unclassified data, those produced, for example, by medical projects.
Suppose a lot of medical imaging data stored in abundance in small local systems can now be uploaded to the cloud and fed from AI. In another case, while extracting and processing the most complex big data sets, ML contributes to data science by automating data analysis with data-informed predictions concerning the high risk of disease.
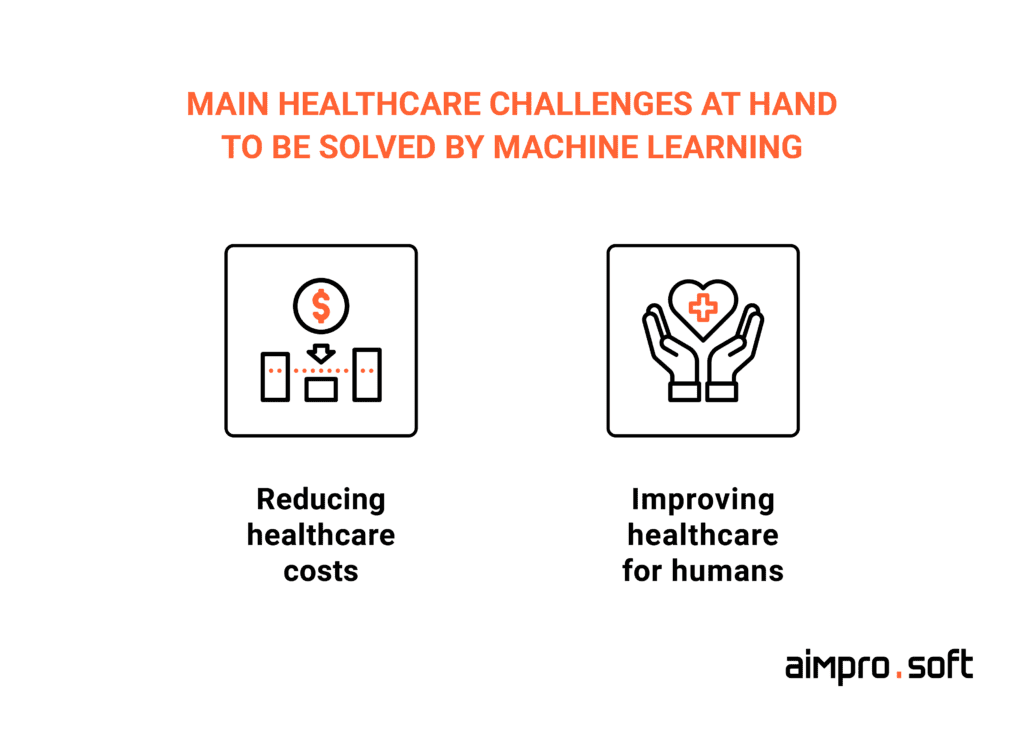
Short years ago healthcare didn’t even expect to be in the center of tech innovations similar to other realms. And yet during January-March in 2020, AI-focused startups in healthcare received almost $1billion of funding projecting the 45% global industry growth by 2026.
Even so, it’s just a scratch on the surface.
According to the electronic library PubMed, the dynamics of the number of publications (over 75 000) on the application of machine learning in medicine in 2011–2021 reflects the exponential growth of the number of studies. This is an ocean of new machine learning project ideas for healthcare use cases for machine learning, taking the sector that has long fallen farther behind to the next level.
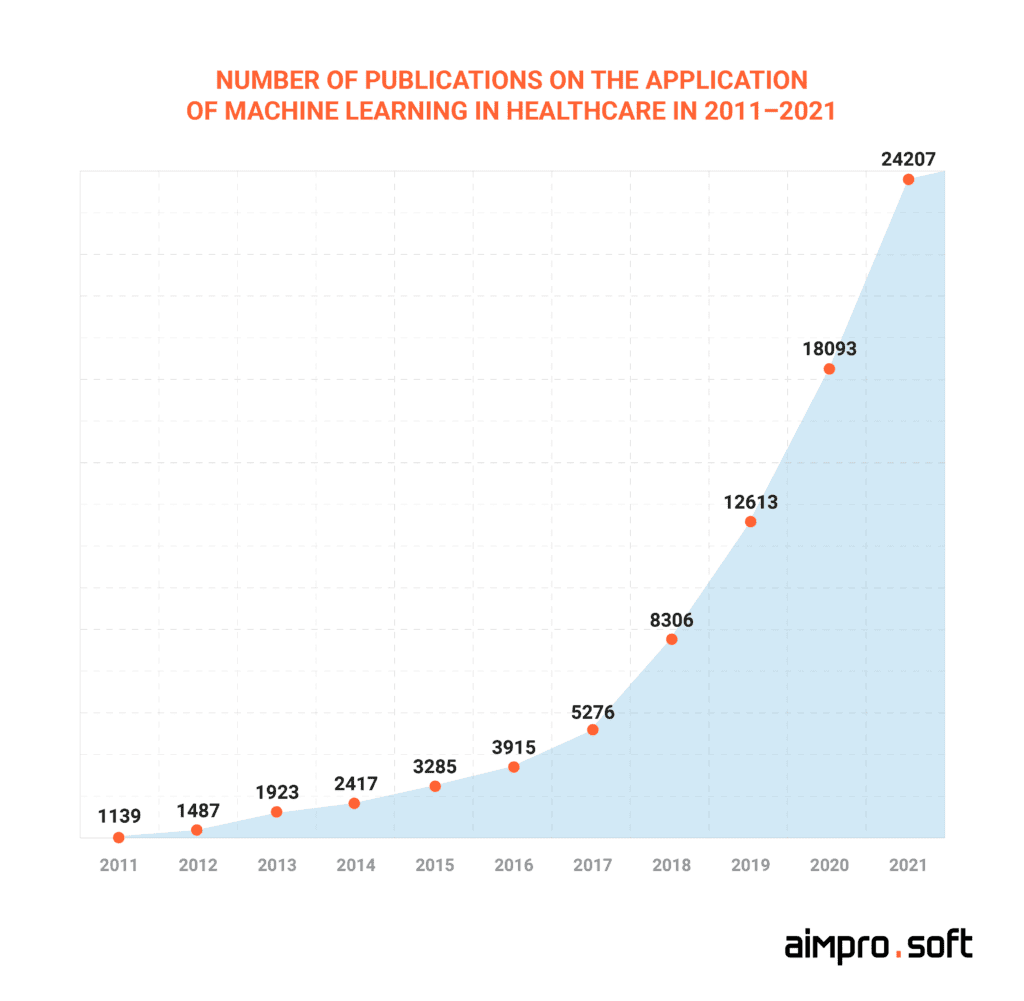
There is another side.
As reported by the National Health Expenditure Accounts (NHEA), the US spent $4.1 trillion for healthcare in 2020. Despite almost omnipresent automation, there are colossal losses that can be saved near-term due to ML algorithms.
As stated by the Accenture report, only virtual nursing assistants can contribute $20 billion back to the sector, thereby making healthcare affordable for any human on the planet in the most remote areas.
Let’s investigate the machine learning healthcare applications by dividing the sphere into three main categories: clinical, pharmaceutical, and administrative.
Machine learning applications in healthcare with clinical validation
Progress boosting software tools are widely embedded in clinical practice in the form of ML-based decision support systems. Extensive incorporation of medical knowledge is able to amplify the expected outcomes and discover new application areas.
Automated diagnosis in seconds instead of days
Amid a diverse set of machine learning methods, there are widespread deep learning projects in healthcare.
Today, computer vision in the medical field is used to determine the state of human health in medical images. Deep learning, a type of machine learning based on artificial neural networks, is able to classify large sets of objects to identify and localize suspicious skin lesions, tumors, internal hemorrhages, brain masses, and more.
Also, magnetic resonance imaging (MRI) benefits from deep learning algorithms like convolutional neural networks regarding scan time reduction. One study fixed a total scan time decrease from standard 279 seconds to 18 seconds.
No matter how hard human tries, it’s beyond their brain’s capacity to accurately process information and deliver specific data about the disease in 3-4 seconds as a computer can.
Caption Health’s machine learning algorithm-based software helps correct capturing and preliminary interpretations of ultrasound images, aiding in preventing heart disease in seconds due to the image recognition mechanism. Paige’s machine learning technology is trained to detect cancer diagnoses from already-analyzed high-resolution images of tissue samples stored in a continuously replenishing database immediately. Thus, this makes clinical decision support more efficient and far less expensive.
However, automated image processing is a drop in the bucket of ML possibilities for health care, by the way.
Conversational interfaces: intake, engagement, and care
More often, a patient’s healthcare journey starts with automated interaction. Often with Google. 43% of people search their symptoms online. A lot of concerns about misdiagnoses rise as a result.
The epoch of talking algorithms improves real-time medical diagnosis assistance, timely disease detection, and integrate smart conversational interfaces into telehealthcare platforms building cost-effective communication with patients full of trust and care.
Natural language processing (NLP) and machine learning techniques enable AI-based chatbots to screen and navigate patients as more natively as possible.
The UK’s National Health Service (NHS) hired a medical chatbot from Babylon Health to diagnose ailments. Having received 1 million calls to their helpline with a $20 cost per one, NHS can save $20 million monthly with the use of AI.
The area of machine learning use cases in the healthcare industry is wide: symptom checker, preliminary diagnosis, intakes, the first contact for primary care, waiting list management, and more. The potential of using AI for patient health cases estimates a 40% rise in outcomes and 50% treatment costs reduction.
ML makes its mark with precision medicine
Since ancient times humans wished to get treated so accurately to respond to their individual peculiarities. Precision medicine of the 21st century is based on the connected data approach using big data and ML in healthcare.
Health records from EHRs and EMRs, medical information from IoT medical devices and sensors, labs, clinics, and other data can be used to form the individual genetic context of a person.
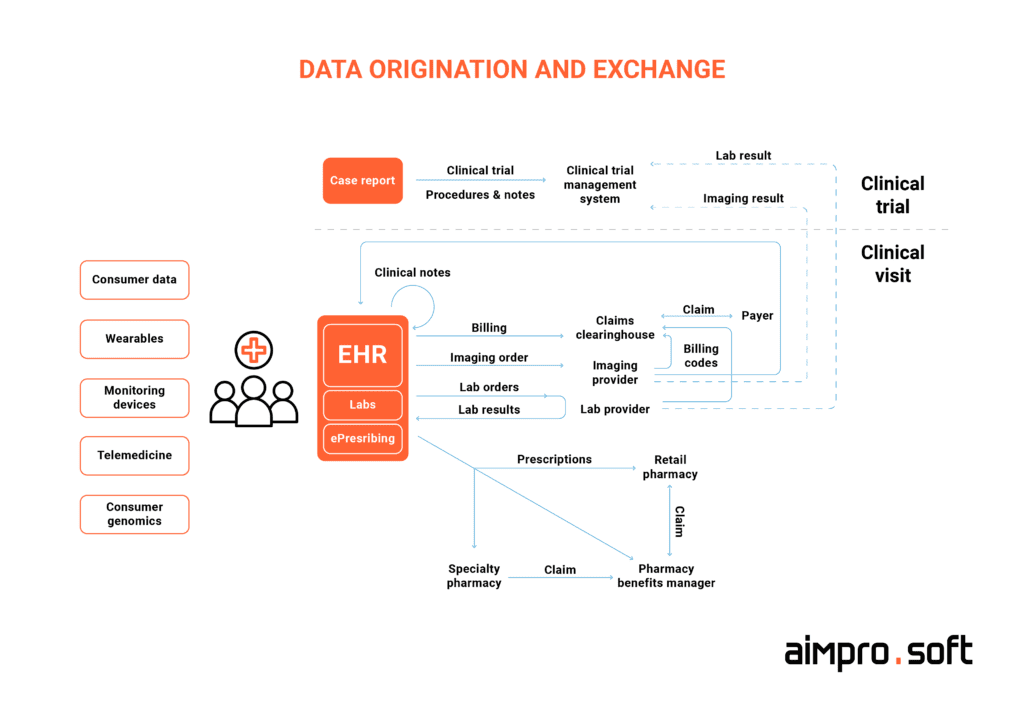
LifeOmic addressed the problem of antiquated healthcare infrastructure with a cloud-based platform. Information about millions of patients collected from electronic medical records, diagnostic images, lab genetic tests, personal health devices is a subject of interest for researchers, scientists, and hospitals in terms of biomarker identification and earlier disease prediction.
Amplion demonstrates another non-trivial technology adoption. Its proprietary business intelligence platform Dx:Revenue precisely matches the test providers and pharmaceutical companies processing with ML over 34 million online sources in real-time, taking precision medicine collaboration to the next level.
Telehealth really blurs the line in the patient care
The success of virtual care access hides some concerns. Yes, we can replace in-person visits and converse via online tools, but patients still need to be examined by a doctor by touch. That’s why telemedicine has been considered rudimentary till now.
One of the uses of machine learning in healthcare is an application to analyze past patient exams to immediately deliver a doctor’s judgment.
Eko went beyond the conventional sense of digital therapeutics. They leveraged machine learning algorithms to detect heart problems with the accuracy of 99% (comparatively to human physicians rate of 70-80%) within their platform equipped with proprietary sensors.
A Biofourmis’s ML-based Biovital Analytics Engine is one of the bright examples of machine learning in healthcare projects that can measure patients’ pain remotely and alarm clinicians to take urgent steps to prevent a fatality.
Another example is Myia, a company powered by predictive analytics and machine learning, which uses sensors to capture vital health stats of diabetes metrics or blood pressure, from patients at home and process data to improve personal care.
Let’s unleash the power of ML algorithms.
CONTACT USPharma R&D: predicting better and earlier with machine learning in healthcare
As in the machine learning use cases in the healthcare industry mentioned above, progress is subject to collaborations. By combining technology and medicine, the world can gain new opportunities and market new products to meet consumer needs with a more accurate market fit.
In pharma R&D, ML algorithms stay on the frontier of reducing the time and cost of developing new life-changing medicine solutions.
Trained models outperform intuition in drug discovery
ML in bio-manufacturing plays a key role in the initial screening of drug compounds as well as predicting how successful the trials can be, taking into account biological factors.
A good example of collaboration is here. Technologists from BenevolentAI and scientists from AstraZeneca joined their efforts to focus on finding out new treatments for idiopathic pulmonary fibrosis (IPF) and chronic kidney disease (CKD). BenevolentAI’ ML algorithms analyze large and highly diverse clinical databases scraping unknown molecular targets to help AstraZeneca discover and develop new, highly responsive drugs.
Another example is Eikon Therapeutics captures data on proteins that move in cells discovering promising drug candidates. ML algorythms derive insights from thousands of data assays running a day.
Intelligent biomarker development
Its meaningful contribution to biomarker development also defines the role of machine learning in the medical field.
BERG, a disruptor in the drug discovery area with its proprietary AI-based Interrogative Biology® Platform with molecular and clinical information, in partnership with Sanofi Pasteur, the vaccines division of pharma Sanofi, enables patients to identify biomarkers and match them with developed influenza vaccines due to AI-modelling. Biology, pharma, technology, and AI intersection allows them to delve into the patient disease profiles to find the best medicines for patients.
Accelerating clinical trials and improving decision-making
The simulation studies held at The University of Texas showed that machine learning methods helped better personalize treatment prescriptions for testees and get more responses among them. ML helps go from randomized to precise picking up candidates, database and document management, discovering subgroup effects, monitoring data quality, and protocol adherence.
Trials entail big data problems that sometimes show a 40% collected data growth per year. Big data paired with ML capabilities can bring a $100 billion value annually, as stated by McKinsey.
Let machine learning predict the efficient research paths.
CONTACT USMachine learning in healthcare: administrative side
The US population spends $1 trillion a year on administration, which is a quarter of all spending in the domain. The health systems of other advanced countries are out of a perfect picture as well. There are around three dozen of voice assistants for doctors globally.
Documenting as fast as speaking
According to the American Medical Association study doctors spend half of their on-duty time dealing with medical records. A lot easier would be to dictate and get notes that appeared in EHR leaving a place for minor human quality control.
AI-backed voice solution from Suki can transcribe real-time doctor-patient conversations with 100% accuracy. NVoq and Augmedix help clinicians fill in patients’ notes in electronic health records systems in seconds. The latter claims saving 2-3 working hours of medics.
NLP, a promising field in machine learning, enables the shift from snailing 40 written to 150 spoken and scribed words per minute, making doctors more performable in their inherent core responsibilities and decreasing spending for the back office.
Have the complaints of medical staff? We revealed here how to eliminate them and better the treatment of the patients.
Data privacy issues solved by machine learning in medical applications
Data privacy concerns continue unabated. Every implementation of every new technology in the healthcare domain is accompanied by strict rules and regulations. And there is a scope for machine learning in healthcare usage.
A principle of remote execution applied in federated machine learning can save data integrity and safety. Since the progress of ML projects in the medical sector depends on data interchanging and training, ensuring data privacy is possible due to sharing deep learning algorithms instead of raw data.
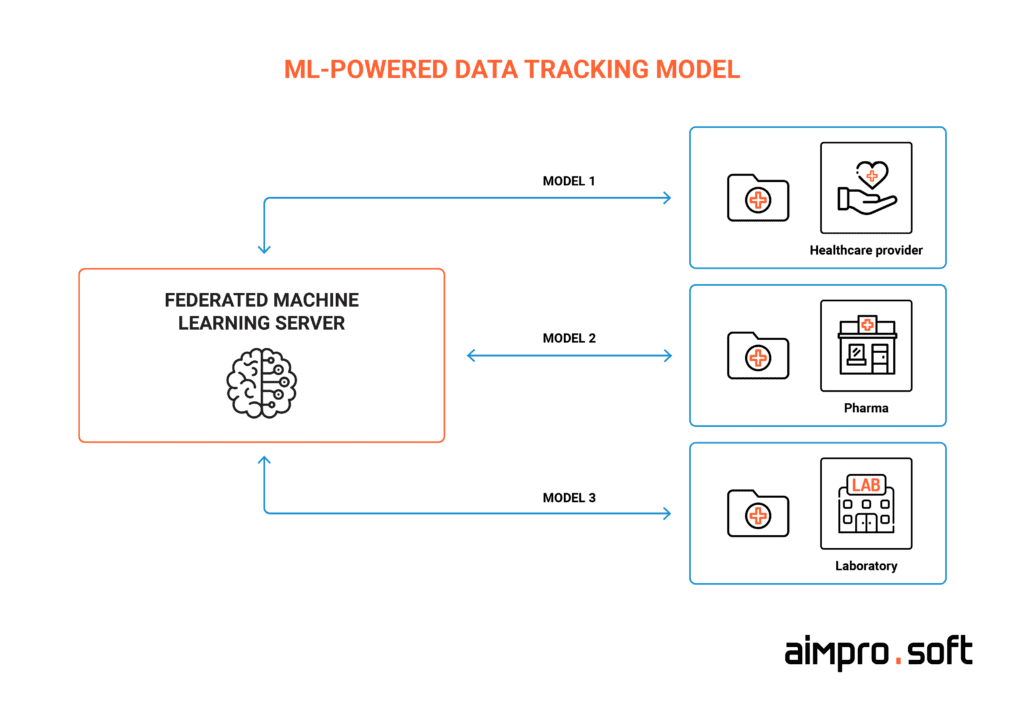
Owkin, an AI-powered biotech unicorn from France, rolled out their proprietary platform enabling data owners to track which model for training their data was used, and their contribution. Certainly, it shows a positive potential for ML in sensitive data management and compliance activities automation.
Seamless data infrastructure
You know better than we do how difficult it is today, no matter what, to get a complete picture of the health status of an individual patient the effectiveness of a new treatment due to a deeply fragmented data landscape.
Datavant, with the help of ML, applied on large datasets, is one of a few that takes health care out of its rigid space. They apply ML to integrated genomic, medical history, and clinical trial data, analytics companies, healthcare providers, EHR, etc. to analyze biological big data in order to collect a complete picture of a person’s health profile.
Medical data is just right for being processed with ML: volumetric, diverse, and frequently updated. ML can blur the boundaries by building a new ecosystem based on digital intuition and automated healthcare analytics.
Regulations and ethics while ML in healthcare adoption
With the active development of technology, it is considered very important to take the necessary measures to regulate the widespread use of technology. The proliferation of AI/ML helped accelerate the adoption of regulations to avoid a last-minute rush while achieving compliance.
Being one of the most progressive states in the world, the FDA (the U.S. Food and Drug Administration) has responded to the rapid medical software development and application of AI/ML technologies with the release of the Action Plan. It reveals regulations on the Software as a Medical Device usage.
Read also how to ensure IoT medical device security for hospitals.
Health Canada and UK’s Medicines and Healthcare products Regulatory Agency (MHRA) teamed up on issuing ten guiding principles regarding the development of the AI/ML best practices.
Moreover, considerations concerning AI/ML usage ethics in medicine bothered researchers from Johns Hopkins University. TriageGo , based on risk algorithms, can assess the state of patients to put in turn for getting emergency aid. The system may escalate into a tool of prevention denial of human rights on racial, gender, or other grounds.
Doing ML projects in the healthcare sector today makes clinical entrepreneurs and scientists think out if their activities threaten patient preference, safety, and privacy. As a software provider, we keep the finger on the regulation pulse to deliver AI/ML compliant solutions.
Read also about the European governmental response to personal data protection in the territory of the EU.
Aimprosoft’s experience of using machine learning in healthcare
Until recently we shared a common understanding of ML cases in healthcare so far. Things changed with a new challenge from our client coming with a brilliant idea of ML application in the area.
Among the variety of machine learning projects in healthcare, medication inventory management in hospitals is in place. Annual spendings for medication inventory management of a single hospital in the Scandinavian countries are quite large. This inefficiency generated an impetus for new products.
A hospital from Norway gave us a request: they asked us to develop an ML solution to streamline the inventory of available pills at the jars by means of stereo images.
Our ML team tackled to develop a particular data evaluation algorithm that allows determining medication bottle pill count. We had to make it possible for healthcare professionals to analyze the consumption of medicines in order to make accurate procurement.
The project is ongoing, so stakeholders expect to get results when the meds can be accurately measured in the bottle down to one pill by means of technology.
Let’s find how ML algorithms can improve your business.
CONTACT USConclusion
Summing up, artificial intelligence technologies are fundamentally changing the global healthcare system, making it possible to radically redesign the medical diagnostics system, develop new medicines, and generally improve the quality of healthcare services while reducing costs for clinics. And ML as a subset of AI takes, you could say, its leading part in the orchestra of digital transformation. Contact us to respond to health care system inefficiencies, taking them as opportunities.
FAQ
What are the benefits of machine learning in healthcare?
Definite advantages are the cost reduction for services and higher quality of care for patients achieved by means of trained ML models that can be created for each particular case.
Are the concerns around ML regarding replacing humans justified?
Not exactly. Let’s say we are a long way off from that. Machine learning stimulates progress in the skills of human professionals. For example, ML can provide radiologists with accurate and quick assistance in diagnostic imaging, improving diagnosis.
What are the most promising applications for ML in healthcare?
Plenty of applications of machine learning in healthcare, examples of high interest besides are big data processing and image recognition. However, algorithms and models are widely used in biotech for AI training, making it quite promising in gaining benefits.